Image of Solar Farm Blended with a Neural Network- (Chesapeake Conservancy/Dr. Michael Evans)
By: Jamal Childs
In a world ever-hungry for renewable energy solutions, the quest to harness the boundless power of the sun has reached a critical point. As the demand for solar energy grew, the need to understand where solar energy was already making its mark and where it could be intentionally placed became paramount. This was the inception of a grand adventure in solar array mapping, guided by the marvels of artificial intelligence (AI) and remote sensing data.

Satellite image of Rockville, MD (2022) – Chesapeake Conservancy/Dr. Michael Evans
At the heart of this undertaking is a revolutionary artificial intelligence (AI) system, a creation born from the amalgamation of deep learning neural networks and reams of remote sensing data about Earth’s surface collected by satellites and planes. The deep learning AI has been a maestro, orchestrating complex information of Earth’s features in its neural network. When the Ai isn’t deciphering codes of information it can be used to map existing solar panels accurately.
This year, the Chesapeake Innovation Center released a new tool that used the power of AI to find solar panels in the Chesapeake Bay Watershed. It can help find solar panels that we do not know about. This can save a lot of time. In comparison, organizations would have to send someone out to survey the land. Being able to show us where solar panels are can also tell us the best places to build them. The AI must learn what it is looking for of course.
“Deep learning refers to a type of predictive model that uses neurons and hidden layers to predict some output from a given set of input variables. This structure is incredibly good at learning complex relationships between input and output variables, which is what is needed to automatically take the information available in remote sensing data and translate it to something…” -Chesapeake Conservancy Senior Data Scientist Michael Evans, Ph.D.

Example of a Deep Learning Neural Network used to train AI – Chesapeake Conservancy/ Dr. Michael Evans
Most solar constructions were found to flourish on agricultural lands. The AI was trained to understand and highlight these areas. So far, the AI found that farmland with the poorest quality soil have been the most likely places for solar panels to be built. This makes the quest for nature positive solar energy more daring. There has to be a balance between conservation and energy production when considering the development of solar panels.
Could building solar panels on optimal sites benefit us in achieving renewable energy goals? Is it worth giving up promising land to develop fields of solar energy? The way land is used is incredibly important for mitigating climate change and reducing emissions. Forests and sustainable farms can be important carbon sinks. We want to make sure we are placing solar panels in ways and in places that preserve the ability of the land to naturally fight climate change.
“There is a whole climate change consideration connected to AI given the massive computing requirements needed to train models, which is why it’s important that cloud computing facilities and data centers be powered by renewables”- Dr. Michael Evans
It is ironic that this tool uses AI to help us understand where solar panels will happen and better prioritize conservation, while at the same time, AI in general is contributing to the very energy consumption needs that raise the demand for putting solar panels in the first place.
The rapid growth of AI has brought about remarkable advancements but also substantial environmental concerns. Ironically, as this AI application, in this case, is helping to prioritize conservation, AI in general is driving a huge demand for energy consumption. One of the primary limitations of AI is its contribution to carbon emissions. As we explored in a Lightning Update earlier this year, the environmental impact largely depends on the source of electricity powering data centers. Data centers are the backbone of AI infrastructure, and their energy consumption can significantly increase carbon emission, particularly when they rely on fossil fuels.
With technology expanding we must learn to adapt with it. The use of AI is becoming more commonplace. It would serve us best if we could find a way to use it strictly for the betterment of conservation in the Chesapeake watershed. As AI continues to evolve and drive innovation, experts are increasingly urging companies to consider the energy consumption and environmental impact of their AI hardware and software development. However, this can be challenging, given the industry’s fast-paced nature and the relentless pursuit of improving AI models’ accuracy and speed.
A critical step towards addressing AI’s carbon emissions is transparency and accountability. There is something to be learned here. In California, where AI-intensive companies like OpenAI and Google are prominent players, Governor Gavin Newsom signed two landmark climate disclosure laws. The first law mandates that companies with global annual revenue exceeding $1 million disclose their carbon emissions from operations by 2026 and their supply chain emissions by 2027. The second law applies to companies with revenues above $500 million and requires them to publish their climate-related financial risk by 2026.
We will have to address issues related with AI, energy, and land use in the Chesapeake watershed. With more tech companies growing and data centers and infrastructure to our region we will need a better understanding at least. We can learn from California’s policies that help us understand the effects these energy needs will have on the land.
As we explored in that earlier Lightning Update, data centers already drive the expanded energy demand in our region. To create a cleaner future, it is important that we strive for 100% renewable energy. Data centers expected in Virginia alone may need 30 gigawatts of energy by 2030. The Piedmont Environmental Council estimates that to generate that amount of energy, it would require about 5% of the land area of Virginia, equivalent to 1.3 Million acres of solar. We are already seeing a huge influx of solar in our region. We need creative solutions to better understand how we can still conserve 30% of our forests, farms, and treasured landscapes by 2030 in the face of the solar demand.

Annapolis Solar Park (2018) – EDF Renewables
AI’s environmental impact is intricately linked to its energy source. Solar power presents a promising solution to mitigate AI’s energy consumption. By harnessing clean, renewable energy from the sun, we can reduce the carbon footprint associated with data centers and AI operations.
Transitioning to solar power not only addresses the environmental concerns but also ensures sustainable AI growth. However, we need to make sure that this solar is put on the land in ways that minimizes the impact of the panels, is compatible with land conservation and our mission to foster collaborative action to conserve culturally and ecologically important landscapes to benefit people, economies and nature throughout the six state watershed.
While AI in general has a big energy footprint, this particular AI application can help us figure out how to mitigate that impact. This relationship underscores the importance of integrating artificial intelligence and solar energy to achieve a more eco-friendly and energy-efficient future for AI technologies.
If you are interested in learning more about this particular AI learning tool, please reach out to Dr. Michael Evans at [email protected].
Image credit:
- Image of Solar Farm Blended with a Neural Network- (Chesapeake Conservancy/Dr. Michael Evans)
- Satellite image of Rockville, MD (2022) – Chesapeake Conservancy/Dr. Michael Evans
- Example of a Deep Learning Neural Network used to train AI – Chesapeake Conservancy/ Dr. Michael Evans
- Annapolis Solar Park – EDF Renewables
Lightning Update is a regular communication of the Chesapeake Conservation Partnership. Any opinions expressed are those of the authors and do not necessarily reflect positions of the Partnership or member organizations.
To share a success story, news, or important event, send your information to:
Support for the Chesapeake Conservation Partnership is provided by:
National Park Service Chesapeake
EPA Chesapeake Bay Program
USDA Forest Service
Pennsylvania Department of Conservation & Natural Resources
Maryland Department of Natural Resources
Virginia Outdoors Foundation
US Fish & Wildlife Service
Chesapeake Conservancy
The Chesapeake Conservation Partnership is co-convened by:


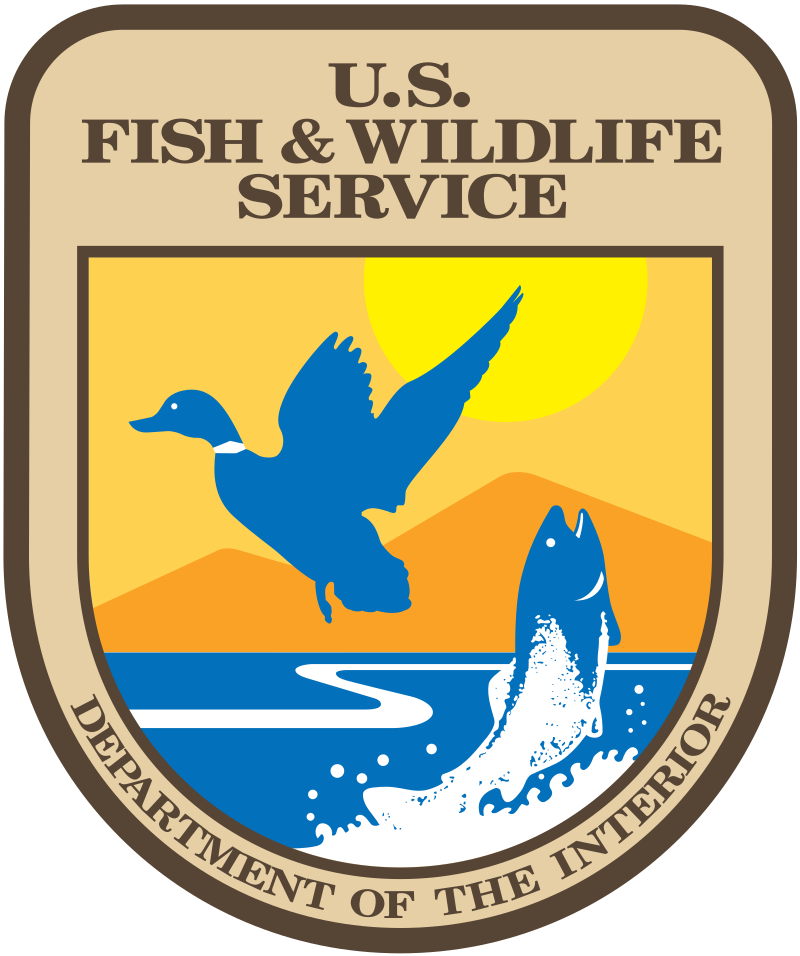